Looking for burst signals
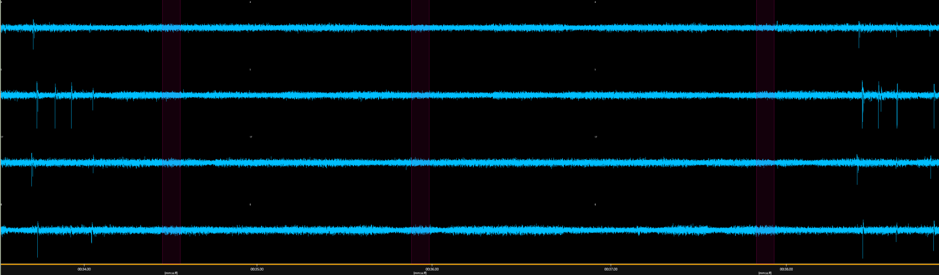
Figure 1. Exemplary spike trains every 4s – BNN from rat cortical stem cells, Bioserver.net, Dec 2019.
One of the most visible features of a functional Biological Neural Network (BNN) is its ability to generate synchronized spikes or burst signals, similar to those observed in living mammal brains.
Once they have reached a sufficient development stage, in particular in terms of connectivity, in vitro neuronal cultures tend to exhibit spontaneous bursts of electrical signals in a way similar to in vivo neural networks. These electrical signals are characterized by oscillatory patterns which are synchronized over large population of neurons and can thus be easily measured to characterize the maturity and health state of the neuronal culture [1] as a pre-requisite towards using it for more advanced experiments, such as direct stimulation.
Bursting activity can be observed in different cultures, such as the rat cortical cultures or hPSC-derived cortical networks generated by differentiation. It is interesting to note that hPSC-derived neuronal networks have been reported to exhibit different patterns compared to rat cortical cultures [2].
For BNN cultures on MEA circuits, these signals may be captured from the MEA electrodes and analyzed with signal processing software to characterize the burst shapes, amplitudes and frequencies. However, in current neuroscience practice, there is no standard analysis method for neuronal burst signal measurements out of MEAs. In the review of Cotteri et alll [3], 8 detectors were compared along 11 formal and experimental properties without identifying a clear winner; a mix of methods may be required for optimal analysis. Manninen et al [4] classifies burst detection methods into three categories: based on spike-data of individual electrodes/neurons, based on population spike-data, and based on global/population firing rate. They also highlight the diversity of models, methods and tools used by the neuroscience community and the difficulty in reproducing experiments due to dependency on the underlying analysis workflows.
The laboratory behind bioserver.net currently conducts various experiments with MEA signal measurements. Ultimately, various options for BNN signal monitoring to match the analysis needs of the end users may be offered, including advanced combinations of sending signal stimuli and measuring BNN signal responses to the stimuli. Do not hesitate to contact us shall you have any specific questions or requirements.
References
- Kirwan et al, “Development and function of human cerebral cortex neural networks from pluripotent stem cells in vitro”, Development 201 142:3178-3187.
- Hyvarinen T. et al, “Functional characterization of human pluripotent stem cell-derived cortical networks differentiated on laminin-521 substrate: comparison to rat cortical cultures”, Nature Research Scientific Reports (2019) 9:17125.
- Cotterill E. et al., “A comparison of computational methods for detecting bursts in neuronal spike trains and their application to human stem cell-derived neuronal networks”, Neural Circuits, Aug 2016
- Manninen T. et al., “Challenges in Reproducibility, Replicability, and Comparability of Computational Models and Tools for Neuronal and Glial Networks, Cells, and Subcellular Structures”, Front. Neuroinform., May 2018