Towards the increase of computing power
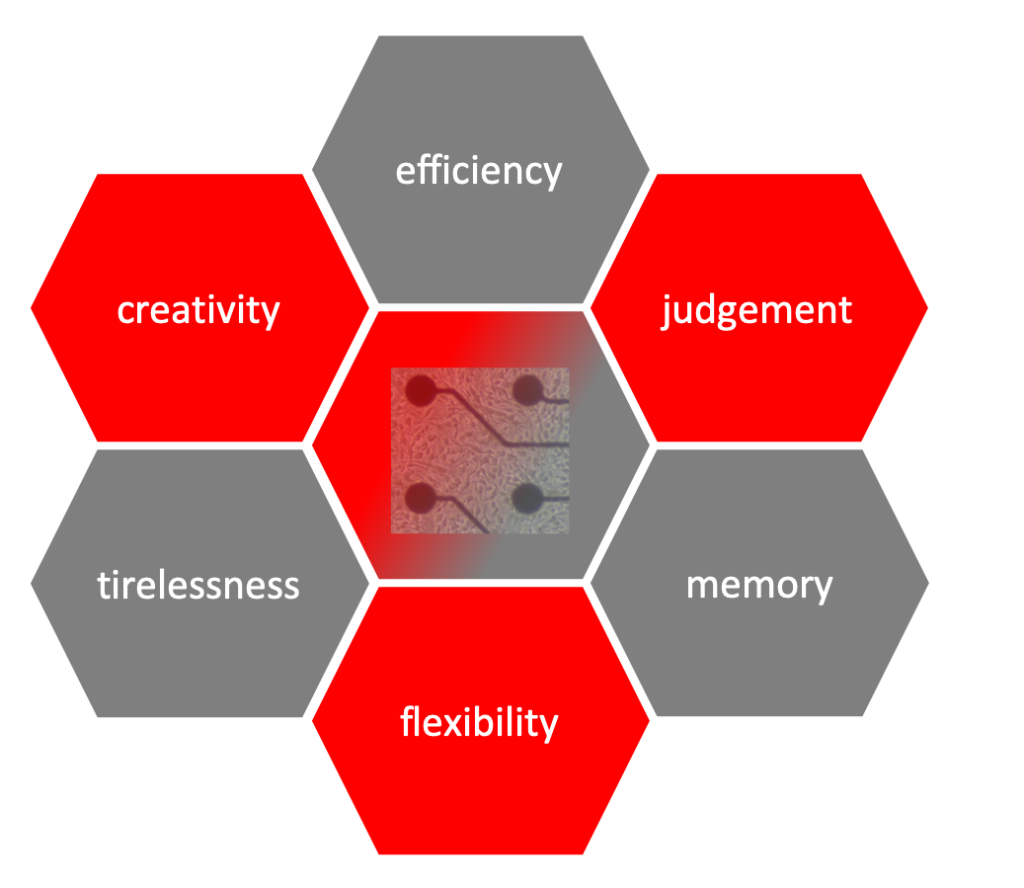
Figure 1: Hardware development can be divided into two directions: mimicking human brain (red) and building artificial systems aimed at efficiency (grey).
Machine learning tools are being developed for over 70 years, however they started to flourish only over the last 20 years. One of the main reasons for this is the fact that sophisticated algorithms need a potent computing power to be executed. Therefore, we can say that the recent progress in hardware development is one of the main reasons for this rapid increase in the development of machine learning tools.
This makes the search for better hardware one of the most important factors which will decide on the speed of the development of Artificial Intelligence.
Software computations are substrate-independent, this means that the same computation can be executed on completely different hardware, as long as it can transform one memory state into another [1]. This gives the possibility for exploration of hardware development into multiple directions, as we do not know which will become the most efficient.
Currently, the best-known computation machine for abstract thinking is the human brain and the best-known machine for fast processing of big volumes of data is a computer. Efforts towards building general artificial intelligence are aimed at building something better by combining these two potent skills.
One of the ways we could look at this is by distinguishing two directions in hardware development:
- towards mimicking the human brain
- towards building something completely different.
Which approach is better?
This is the same type of question which was asked before we build planes: is it better to mimic nature, by building artificial birds or should we approach the problem from a different angle? In the case of planes, the second approach worked better, however the distinction between mimicking nature and building something completely different is not that clear. We use completely different materials to build planes, but aerodynamics is inspired by what we see in nature. We could say that, for the plane building, we use different materials but similar principles for design. So perhaps a right combination of these two approaches gives the best results?
It is hard to say if a similar approach will work for designing better hardware to facilitate the development of general AI.
We couldĀ again, divide the problem into two main directions, reflecting the distinction between mimicking the nature versus a completely different approach.
Development of material used to build the hardware could be seen as:
- a question for material sciences, to search for better materials allowing faster signal processing
- a question for biology, how to use the best natural signal processing unit we know so far, a neuron.
Further, we could also apply an analogous distinction for the design of the hardware by searching for:
- combination of a large number of identical units
- a whole brain emulation [2] or similar, by building a complex structure with units which are diverse but complementary.
From the neuroscience perspective, mimicking the human brain seems not to be a feasible approach in the nearest future, given the complexity of its structures and our little understanding of how it really works. Building 1:1 prototype using advanced imaging data and 3D printing could potentially be an option if we could one day print living cells. For the moment, a more simple approach could be to use a bunch of living neurons as a signal processing unit [3] and let it grow on a surface which can transmit its signals further to allow a connection to an interface.
This is an interesting combination of mimicking nature (for the materials used) and building something completely new out of it (in terms of design). Quite the opposite way, as we build planes, but perhaps it will work that way this time?
Literature:
- Tegmark, Life 3.0: Being Human in the Age of Artificial Intelligence. , 2017. Print.
- Bostrom, Superintelligence: Paths, Dangers, Strategies, Oxford University Press, Inc. 198 Madison Ave. New York, NYUnited States, 2014
- Hu et al,, 2014, A neuron as a Signal Processing Device.