Understanding the spikes of the neurons
What is a neuronal spike?
Neuronal spike is an electrical signal generated by a neuron. Neuronal cells have a membrane potential which means that the electric charge (expressed in voltage) is not the same on both side of its membrane. This is due to the difference in ion concentrations on external versus internal side of the membrane.
When a signal is transmitted through the neuron, practically that means that ions are travelling across the membrane, parallel to the direction of the changing electrical signal along the membrane.
The initial trigger for neuronal spiking is an electric or chemical (e.g. ion or neurotransmitter) signal changing neuronal membrane potential. If the change is large enough (above a certain threshold), the signal will be propagated to the other side of the cell.
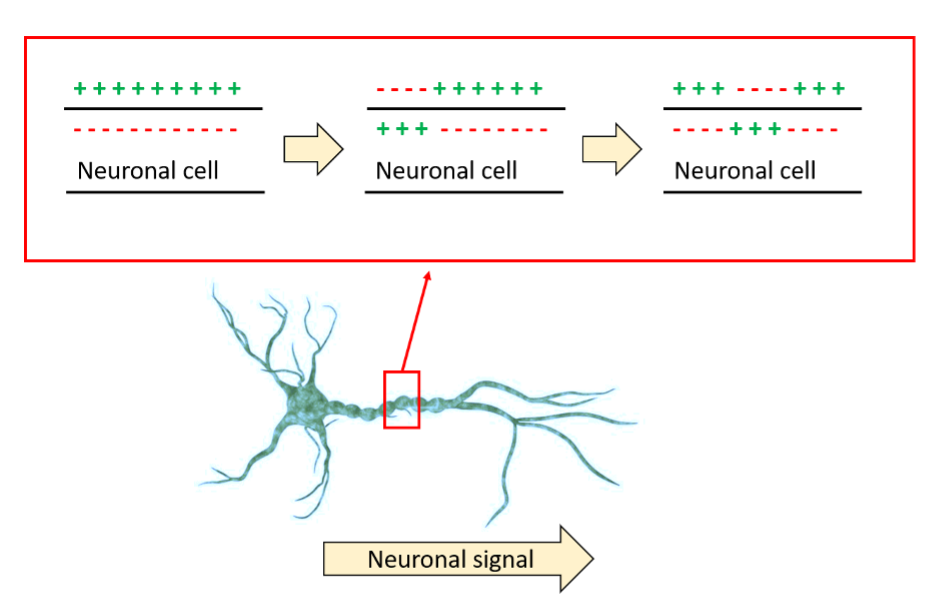
Fig 1. A schematic overview of how ion crossing the neuronal membrane allow the transmission of an electric impulse through this membrane.
Below is a typical shape of a voltage change versus time in the neuronal membrane during the occurrence of one single spike:
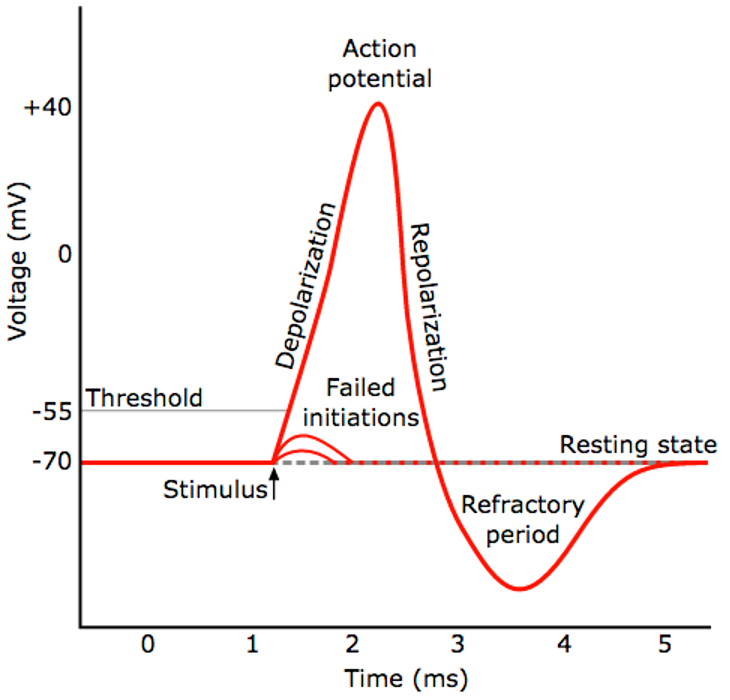
Fig 2. An example of a neuronal membrane voltage changes over time during a single spike. Once a stimulus passes the threshold value, a sharp change in voltage (action potential) occurs. This is followed by a short refractory period before the membrane voltage comes back to the resting state, in other words, ion concentrations come back to the resting state on both sides of the membrane. Image source: https://www.kurzweilai.net/ibm-scientists-emulate-neurons-with-phase-change-technology
Multiple spikes can be depicted as spike trains, indicating the frequency of peaks occurring over time. In our lab, we register spikes in real time, live demonstration is available on our website: bioserver.net/live/.
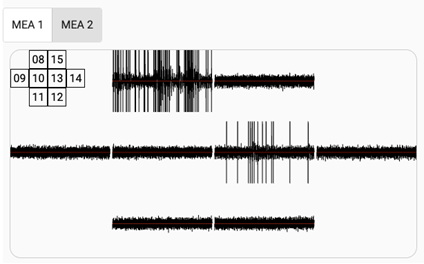
Fig 3. An example of Biological Neural Network activity measured on 20th Aug 2020. In the upper left corner we have schematic view of the multielectrode plate layout, on which neurons are living, with the numbers indicating the electrode ID.
Neuronal spikes can be recorded in two main ways:
- Without external electrical stimulus
- During unilateral communication, when only recording of the signal from the neuron to the external device is performed.
- This is called ‘spontaneous spiking activity’
- With external electrical stimulus
- Bilateral communication, when we both register the spiking activity of the neurons and provide external stimuli to them.
- In this case we can statistically demonstrate relationships between external stimulus and the occurrence of the spikes [Pelt et al. 2008]. This is the first step to design signals which can trigger desired response of the neuron in vitro.
We can distinguish 2 main types of neuronal spikes:
- Instant answer: the stimulation leads neuron(s) to fire spike(s), typically less than500 ms after stimulation.
- Long-term potentiation (LTP): the spontaneous firing rate is modified via a feedback loop during a period of several hours.
We can design electrical stimulus by adjusting the voltage and duration of the signal, for example:
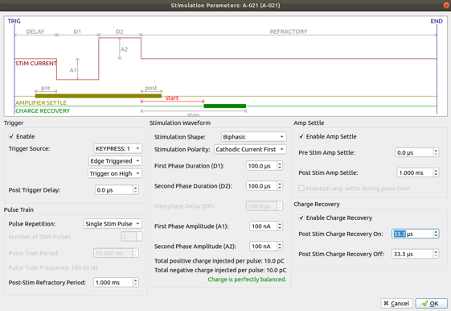
Fig. 5. An example of a design of an electrical signal to stimulate neuronal culture.
A consistent model for online recording and stimulation of neuronal activity opens opportunities for wider studies on neuronal signalling.
Understanding the meaning of the neuronal spikes.
We can distinguish two approaches to this problem:
- Stochastic approach: here we assume that the neuronal spiking to a given stimulus is variable and can be analysed statistically. Different neuronal spike responses to the same input signal is considered as a noise [2].
- Predictive homeostasis: here we assume that the reaction to the stimulus depends not only on the triggering signal, but also on the current state of the neuron (e.g. its membrane homeostasis). Different neuronal spike response to the same input signal is not considered as a noise, but is believed to reflect the current state of the neuron [3].
Both approaches are being used in neuroscience to explain the meaning of neuronal spikes [2, 3]. Both ways of thinking have some advantages and disadvantages. Stochastic approach is more feasible for the experimental analysis, however it is also a simplified view of the problem. Predictive homeostasis approach could be more informative but also requires more elaborate experimental setup, which is not always feasible to apply, especially in vivo. However, this way of thinking, reminds us of the importance of ensuring stable health of the cells in culture in order to ensure the reproducibility of the experiments.
Whether on a statistical or more mechanistic level, we are always trying to work with two main phenomena:
- How external stimuli trigger the neuronal spikes (e.g. which electrical signals trigger which spikes)
- How neuronal spikes trigger organism response/output device response (e.g. muscle movement in vivo or electrical signal received by a device in vitro)
Being able to control these complex relationships allows us designing systems which behave in a particular way, for example to further improve the design of:
- Specific electrical signals triggering the cells to process the information in neurocomputer [4]
- Prosthetic devices [5, 6, 7]
- Neurorehabilitation tools, such as video games used for motor retraining and rehabilitation designed to enhance neuronal plasticity [8, 9].
Literature
- Pelt J., Vajda I., Wolters P., Ramakers G., van Ooyen A. (2008) Effect of Low-Frequency Stimulation on Spontaneous Firing in Cultured Neuronal Networks. In: Wang R., Shen E., Gu F. (eds) Advances in Cognitive Neurodynamics ICCN 2007. Springer, Dordrecht. https://doi.org/10.1007/978-1-4020-8387-7_11
- Aljadeff J, Lansdell BJ, Fairhall AL, Kleinfeld D. Analysis of Neuronal Spike Trains, Deconstructed. Neuron. 2016;91(2):221-259. doi:10.1016/j.neuron.2016.05.039 link: https://pubmed.ncbi.nlm.nih.gov/27477016/
- Fiorillo CD, Kim JK, Hong SZ. The meaning of spikes from the neuron’s point of view: predictive homeostasis generates the appearance of randomness. Front Comput Neurosci. 2014;8:49. Published 2014 Apr 29. doi:10.3389/fncom.2014.00049 link: https://pubmed.ncbi.nlm.nih.gov/24808854/
- Ruaro ME, Bonifazi P, Torre V. Toward the neurocomputer: image processing and pattern recognition with neuronal cultures. IEEE Trans Biomed Eng. 2005;52(3):371-383. doi:10.1109/TBME.2004.842975 link: https://pubmed.ncbi.nlm.nih.gov/15759567/
- Özmert E, Arslan U. Retinal Prostheses and Artificial Vision. Turk J Ophthalmol. 2019;49(4):213-219. doi:10.4274/tjo.galenos.2019.44270 link https://pubmed.ncbi.nlm.nih.gov/31486609/
- Bin Sun, Tengyue Li, Kai Xia, Qi Zeng, Tianzhun Wu, Humayun MS. Flexible microelectrode array for retinal prosthesis. Conf Proc IEEE Eng Med Biol Soc. 2017;2017:1097-1100. doi:10.1109/EMBC.2017.8037019 link: https://pubmed.ncbi.nlm.nih.gov/29060066/
- Normann RA, Maynard EM, Rousche PJ, Warren DJ. A neural interface for a cortical vision prosthesis. Vision Res. 1999;39(15):2577-2587. doi:10.1016/s0042-6989(99)00040-1 link: https://pubmed.ncbi.nlm.nih.gov/10396626/
- Samuel GS, Oey NE, Choo M, et al. Combining levodopa and virtual reality-based therapy for rehabilitation of the upper limb after acute stroke: pilot study Part II. Singapore Med J. 2017;58(10):610-617. doi:10.11622/smedj.2016111 link: https://pubmed.ncbi.nlm.nih.gov/27311739/
- 9. Reinkensmeyer DJ, Burdet E, Casadio M, et al. Computational neurorehabilitation: modeling plasticity and learning to predict recovery. J Neuroeng Rehabil. 2016;13(1):42. Published 2016 Apr 30. doi:10.1186/s12984-016-0148-3 link https://pubmed.ncbi.nlm.nih.gov/27130577/